At the Center for Connected Learning and Computer-Based Modeling (Uri Wilensky, Director), we were: (a) building agent-based computer models as learning tools for students to simulate scientific, mathematical, and social phenomena; and (b) analyzing emprical data from our design experiments to develop theoretical models of cognition, teaching, and learning. These theoretical models were by and large expressed in diagrams and words. One day it occurred to us that we could combine these two activities — building models, modeling behavior — in a new way. And so we created agent-based virtual models to simulate our diagram-based theoretical models. At first, we built the “I’m Game!” model to compare predictions from Piagetian and Vygotskian models of learning. This led to a welcome pushback from sociocultural theorists Mike Cole and Jim Levin who raised the gauntlet and improved on our model, demonstrating how the zone of proximal development wins big time… But then, inspired by Paulo Blikstein’s emerging ideas of bifocal modeling, we decided to use real and simulated data side by side. This led to a series of computational-modeling studies of Piagetian interviews as well as classroom interactions, where we ran actual and simulated data in parallel so as to tweak the theoretical assumptions underlying our simulations.
Thus at the CCL this direction of research tackled distributed-learning theoretical models from a complexity-studies perspective to frame the design and implementation of agent-based models and their extensions that support participatory simulations in mathematics classrooms. At its broadest, this line of research uses agent-based modeling to study and develop theoretical models of individual group learning.
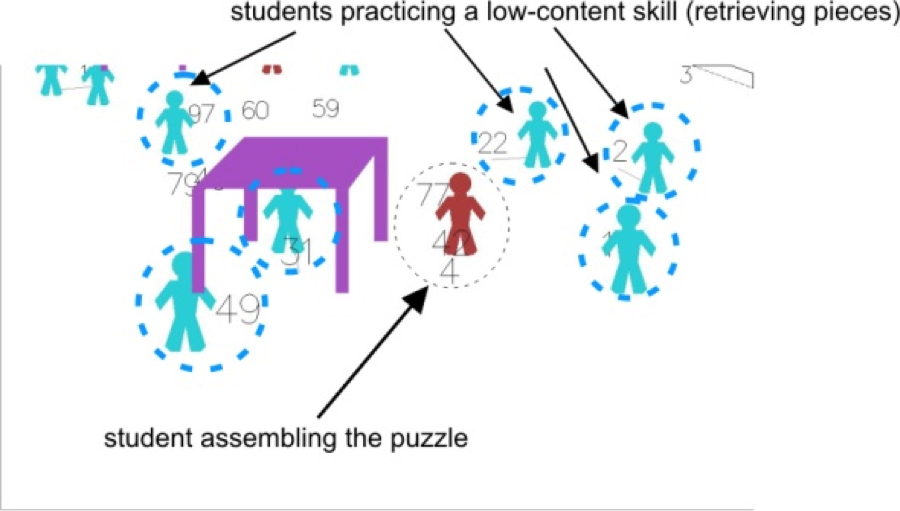
Publications
Blikstein, P., Wilensky, U., & Abrahamson, D. (2009). Towards a framework for cognitive research using agent-based modeling and complexity sciences. In M. Jacobson (Symposium Chair), M. Kapur (Organizer), & N. Sibelli (Discussant), Complexity, learning, and research: Under the microscope, new kinds of microscopes, and seeing differently. Paper presented at the annual meeting of the American Educational Research Association, San Diego, April 13-17.
OPENING PARAGRAPH: Complexity sciences and agent-based modeling has been increasingly used by scientists to study a wide range of phenomena such as the interactions of species in an ecosystem, the collisions of molecules in a chemical reaction, or the food-gathering behavior of insects (Bonabeau, 1999; Wilensky & Reisman, 2006). Such phenomena, in which the elements within the system (molecules, or ants) have multiple behaviors and a large number of interaction patterns, have been termed complex and are collectively studied in a relatively young interdisciplinary field called complex systems or complexity studies (Holland, 1995). Typical of complex phenomena is that the cumulative (‘aggregate’) patterns or behaviors at the macro level are not premeditated or directly actuated by any of the “lower-level” micro elements. For example, flocking birds do not intend to construct an arrow-shaped structure (Figure 1), or molecules in a gas are not aware of the Maxwell-Boltzmann distribution. Rather, each element (“agent”) follows its local rules, and the overall pattern arises as epiphenomenal to these multiple local behaviors—the overall pattern emerges. In the mid-nineties, researchers started to realize that agent-based modeling could have a significant impact in education (Resnick & Wilensky, 1993; Wilensky & Resnick, 1995). For instance, to study the behavior of a chemical reaction, the student would observe and articulate only at the behavior of individual molecules — the chemical reaction is construed as emerging from the myriad interactions of these molecular “agents.” Once the modeler assigns agents their local, “micro” rules, the model can be set into motion and the modeler can watch the overall patterns that emerge.
Blikstein, P., Abrahamson, D., & Wilensky, U. (2008). The classroom as a complex adaptive system: an agent-based framework to investigate students’ emergent collective behaviors. In G. Kanselaar, J. v. Merriënboer, P. Kirschner, & T. d. Jong (Eds.), Proceedings of the Eighth International Conference of the Learning Sciences (ICLS2008) (Vol. 3, pp. 12-13). Utrecht, The Netherlands: ISLS.
ABSTRACT: This study applies agent-based modeling methodology to investigate individual and social factors underlying inequitable participation patterns observed in a real classroom in which an experimental collaborative activity was implemented. We created agent-based simulations of simplified collaborative activities and qualitatively compared results from running the model with the classroom data. We found that collaboration pedagogy emphasizing group performance may forsake individual learning, due to preference for shortterm group efficacy over individual long-term learning. The study may inform professional development and pedagogical policy.
Blikstein, P., Abrahamson, D., & Wilensky, U. (2008, March). Groupwork as a complex adaptive system: A methodology to model, understand, and design classroom strategies for collaborative learning. Paper presented at the annual conference of the American Education Research Association, New York, March 24-28.
OPENING PARAGRAPH: Agent-based modeling (ABM) has been increasingly used by scientists to study a wide range of phenomena such as species in an ecosystem or molecules in a chemical reaction (Bonabeau, 1999; Wilensky & Reisman, 2006). Such phenomena, in which the elements within the system have multiple behaviors and a large number of interaction patterns, have been termed complex and are studied in the field called complex systems (Holland, 1995). Typical of complex phenomena is that the cumulative (‘aggregate’) patterns at the macro level are not premeditated by the “lower-level” micro-elements. For example, flocking birds do not intend to construct an arrow-shaped structure (Figure 1), or molecules are not aware of the Maxwell-Boltzmann distribution. Rather, each element (“agent”) follows its local rules, and the overall pattern emerges as epiphenomenal to these multiple local behaviors. In the mid-nineties, researchers realized that ABM could have a significant impact in education ((Resnick & Wilensky, 1993; Wilensky & Resnick, 1995). To study the behavior of a chemical reaction, students would observe and articulate only at the behavior of individual molecules — the chemical reaction is construed as emerging from the myriad interactions of these molecular “agents.”
Abrahamson, D., Blikstein, P., & Wilensky, U. (2007). Classroom model, model classroom: Computer-supported methodology for investigating collaborative-learning pedagogy. In C. Chinn, G. Erkens, & S. Puntambekar (Eds.), Proceedings of the Computer Supported Collaborative Learning Conference (CSCL) (Vol. 8, Part 1, pp. 46-55). NJ: Rutgers University. CD ROM.
ABSTRACT: We have been exploring the potential of agent-based modeling methodology for social-science research and, specifically, for illuminating theoretical complementarities of cognitive and socio-constructivist conceptualizations of learning (e.g., Abrahamson & Wilensky, 2005a). The current study advances our research by applying our methodology to pedagogy research: we investigate individual and social factors underlying outcomes of implementing collaborative inquiry classroom practice. Using bifocal modeling (Blikstein & Wilensky, 2006a), we juxtapose agent-based simulations of collaborative problem solving with real-classroom data of students’ collaboration in a demographically diverse middle-school mathematics classroom (Abrahamson & Wilensky, 2005b). We validate the computer model by comparing outcomes from running the simulation with outcomes of the real intervention. Findings are that collaboration pedagogy emphasizing group performance may forsake individual learning, because stable division-of-labor patterns emerge due to utilitarian preference of short-term production over long-term learning (Axelrod, 1997). The study may inform professional development and pedagogical policy.
Abrahamson, D., Wilensky, U., & Levin, J. (2007, April). Agent-based modeling as a bridge between cognitive and social perspectives on learning. Paper presented at the annual meeting of the American Educational Research Association, Chicago, IL, Chicago, IL.
ABSTRACT (Symposium): The symposium presents exemplars of the potential power of complexity-studies methodology, embodied in agent-based modeling, for engaging in research on psychological phenomena involving individual learners in social contexts. Working in the NetLogo environment (Wilensky, 1999), we explore the debate between Piagetian and Vygotskiian accounts of learning, the development of student reasoning on Piagetian conservation tasks, and student’s implicit argumentation strategies in science inquiry. Also, we reflect on the artifacts of ABM as mediators of distributed research effort. ABM lenses could enable education researchers to explore, articulate, and share intuitions we have struggled to study and express — that individual intelligent behavior emerges from multicomponential cognitive interactions and, one “level up,” that individuals and communities are interdependent through myriad dynamic reciprocities.
ABSTRACT (paper): This paper is a proof-of-existence empirical paper. That said, it is also a methodological paper. The methodology is agent-based modeling, a computer-supported mode of inquiry into complex phenomena, such as weather fronts, market fluctuations, or participation patterns in a middleschool mathematics lesson. We have previously shown that agent-based simulation can express theoretical models of learning (Abrahamson & Wilensky, 2005; see also Smith & Conrey, 2007). In that paper, we claimed that a promising attribute of simulation-based research into learning is that it fosters scholarly critique and engaging collaboration. It is that claim that is herein proven to stand. The proof lies in the collaborative criticism offered by the third author, Jim Levin, to the first two authors, Dor Abrahamson and Uri Wilensky, concerning their agent-based simulation of learning, which was first presented at the 2005 annual meeting of the Jean Piaget Society and was then made available online, along with its underlying computational procedures that were laid out for scrutiny. The 2005 paper explicitly invited fellow researchers to critique the simulation and possibly modify it so as to accommodate their own perspectives and possibly enable their own investigations. Indeed, the critique received from Levin took a unique form—he improved the computer-based model such that it better simulates the target constructs. Such coconstructive critique, we argue, is a hallmark of the promise of agent-based modeling. So we submit that this proof of existence, if anecdotal validation, may be a harbinger of a new mode of research in the learning sciences and beyond—a mode that builds on constructionism (Papert, 1991): constructionist collaboration.
Blikstein, P., Abrahamson, D., & Wilensky, U. (2006, June).Minsky, mind, and models: Juxtaposing agent-based computer simulations and clinical-interview data as a methodology for investigating cognitive-developmental theory. Paper presented at the annual meeting of the Jean Piaget Society, Baltimore, MD.
ABSTRACT: We discuss an innovative application of computer-based simulations in the study of cognitive development. Our work builds on previous seminal contributions to field, in which theoretical models of cognition were implemented in the form of computer programs in attempt to predict human reasoning (Newell & Simon, 1972; Rose & Fischer, 1999). Our computer model can both be a useful vehicle to illustrate the Piagetian theoretical model or to simulate it departing from clinical interview data. We focused in the Piagetian conservation experiment, and collected and analyzed data from actual (not simulated) interviews. The interviews were videotaped, transcribed, and coded in terms of parameters of the computer simulation. The simulation was then fed with these coded data. We were able to perform different kinds of experiments: Playback the interview and the computer model side-by-side, trying to identify behavior patterns; Model validation: investigate whether the child’s decision-making process can be predicted by the model. We conclude that agent-based simulation, activated alongside real data, offers powerful methods for exploring the emergence of self-organized hierarchical organization in human cognition. We are currently exploring the entire combinatorial space of all hypothetical children’s initial mental states and activating the simulation per each of these states. From that perspective, our data of real participants become cases out of the combinatorial space.
Abrahamson, D., & Wilensky, U. (2005, June). Piaget? Vygotsky? I’m game!: Agent-based modeling for psychology research. Paper presented at the annual meeting of the Jean Piaget Society.
ABSTRACT: We discuss agent-based models (ABM) as research tools for developmental and social psychology. “Agents” are computer-based entities, e.g., “people.” The modeler assigns the agents real-world roles and rules, conducts simulation experiments in which the agents follow their rules, and observes real-time data. Agent-based models have some properties that can be very useful to psychology. Agent-based models are more dynamic and more expressive as compared to diagrammatic models. Also, simulations afford immediate feedback on the validity of the models. Agent-based modeling is useful for understanding complex phenomena, e.g., the dynamics of multiple individual learners interacting with their peers and with artifacts in their environment and the emergent group patterns arising over time from these multiple interactions. We demonstrate an agent-based simulation that we designed as a “thought experiment” that can shed light on the ongoing debate between two theories of learning, constructivism and social constructivism. The process of building the simulation and embodying these learning theories in explicit rules is an example of how agent-based modeling may help researchers in honing their theories. When “running” the models, unexpected consequences arise, and this leads to successive refinement of theory.